Server Environments
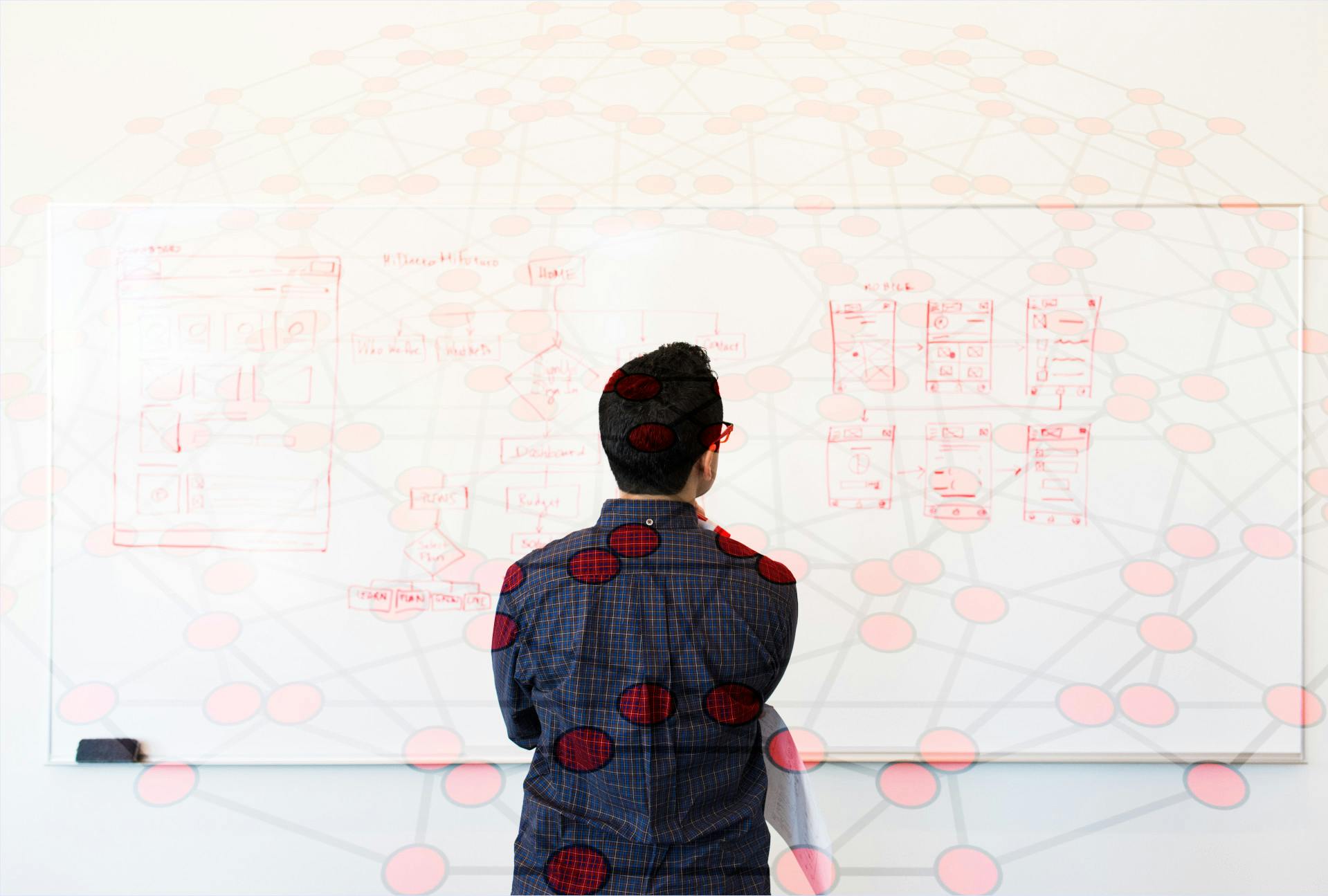
When maintaining large software systems, you will likely have multiple environments with names like Prod, Staging, Dev, Eval, UAT, Daily, Nightly, or some remix of these names. To distinguish this type of environment from the dozen other things in software development that we give that same name, these are often formally referred to as Deployment Environments.
One question I’ve never been asked directly is: “What is an Environment?” This is surprising; because not understanding what Deployment Environments actually are is one of the most common pitfalls I see in my day-to-day work.
Read more →